Last year, 2023, was certainly the year that large language models (LLMs) and generative pre-trained text (GPT) went mainstream. Every conference I attended had a plethora of presentations on AI. Marketing material was saturated with AI-powered this and that or AI-ready technology, which left me scratching my head trying to figure out what that really meant. All at once many of the tools I use daily had a button to “generate heading,” “generate text,” and the worst…”generate blog.”
Generative Text
I experimented a lot with the tools I had available. While I’m getting better at prompts for generative text, I’m certainly not ready to use a GPT model to write a blog post about DCL’s specific services and why our processes, people, and tools are exceptional. I did try! ChatGPT followed my prompt to draft a:
“2500-word blog post about the importance of the Process Data Module in S1000D. Detail why the Process Data Module is important for an individual organization as well as the larger aerospace and defense industries. Integrate keywords such as xml conversion, structured content, s1000D, process data module.”
When I shared the sample blog post with DCL’s S1000D experts, which took all of 3 seconds for ChatGPT to compose, I received the quickest response from one of my colleague: “Do not post. This is utter crap.”
I continued blog writing experiments but none of the generated text was something I wanted to post. In fact, I’m starting to discern text that’s been generated from GPT from human-created content. There is a certain banal quality to generated text…a true lack of personality.
Generative Images
I did achieve success generating images with DALL-E and Adobe Express. The generated graphics saved me loads of time and money searching for images from online market places and editing them to suit my needs. I feel confident in drafting and fine-tuning my text-to-image prompts:

Generative fill experiments were not as successful. When I mixed a real photo with “AI-powered” generative fill to portray a scene that did not exactly exist things got murky. It's pretty clear in the following image that this is fake. I was on stage speaking but the streamers and 6-people deep audience were not there:
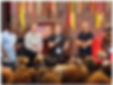
AI Acceptable Usage Policy
This year DCL’s first initiative has been to create an “AI Acceptable Usage Policy” for staff. As an ISO-certified company that has been in business for well over 40 years, it’s mission critical that we ensure staff understand the acceptable and responsible use of artificial intelligence (AI) technologies within DCL. The policy aims to ensure the ethical, legal, and secure utilization of AI tools and services provided by third-party vendors. It also addresses any external tools used by DCL employees, contractors, and systems that involve sending data externally.
DCL’s IT maintains a list of approved tools for staff to use and we have continuous education at company meetings that review data confidentiality, data safety, data retention, and the approval process to experiment with any new tools.
AI is Not New Technology for DCL
While AI-everything blew up last year, it’s not a new technology for DCL. If you’ve chatted with me about artificial intelligence and machine learning one of the first things I like to remind people is that optical character recognition (OCR) is the original machine learning.
Optical character recognition is the process of converting images of typed, handwritten, or printed text into machine-encoded text. The genesis of OCR is older than you might think! The concept traces back to the late 1920s when the Austrian engineer Gustav Tauschek obtained the first patent for his “Reading Machine.”
When it originated, OCR was a mechanical process. Tauschek’s Reading Machine was engineered with gears, mechanisms, and photodetectors that used the input of printed text and generated the output of that text printed on paper.

OCR is taken for granted today as OCR services have become commoditized and software such as Adobe Acrobat will automatically apply OCR to a document. However, early OCR systems needed to be trained with images of each character and worked on one font at a time. For this reason, we like to say that at its core, OCR is an early example of machine learning.
Today, DCL employs a number of AI-related technologies into our systems, services, and tools. I asked some colleagues to share their take on the AI explosion and some of the more exciting ways we employ AI:
People use the overarching term “artificial intelligence” but are not really clear on the nuances between AI, ML, NLP, etc. Could you give a quick primer on the key terms we hear and what they mean?
Rich Dominelli, Systems Architect, DCL
Let's talk about what we mean by artificial intelligence. Artificial intelligence is any time we're trying to use computers to emulate the way human thought works, to perform things that are typically hard for computers to do. To understand natural language, or interpret vision, or make decision processes in a fashion that's much closer to the way that people think.
Back in the day, Ray Kurzweil when he was working on OCR, did a lot of modeling for how the human brain processes vision and how it interprets characters. And that was kind of, I don't want to say the foundation of modern AI, but it was a big step forward in AI. Machine learning on the other hand is taking neural networks and basically building a statistical model. It's a branch of artificial intelligence. It's a subset of it, but it's basically using large statistical models to infer results based on previous training. Deep learning is a subset of it. Again, we have these large statistical models. Sometimes we have emergent thought processes, and these statistical models have grown to the point where it's getting hard to understand what they're doing under the covers.
And then we have natural language processing where we're trying to have computers understand the nuances of unstructured data and speech and prose. The English language compared to computer language or honestly any language compared to computer language is much less rigid in its interpretation. It's much more nuanced. There's a lot of context clues that are going on. So, these are things where we're trying to have the computer understand exactly what's written.
Predictive engines like ChatGPT, Bard, and others have really captivated attention over the past year. Can you share how DCL has been using AI-related technologies over its history since 1981?
Mike Gross, CTO, DCL
We’ve employed Computer Vision (CV) since the beginning of DCL around 1981. The technology dates back to the late 1950s and early 1960s at NIST. CV is a type of AI that allows machines to interpret and make decisions based on visual data and uses algorithms and models to extract meaningful information from images or videos. It involves tasks such as image recognition, object detection, and scene understanding, allowing computers to "see" and comprehend the visual content of their surroundings. People are likely familiar with CV from applications like facial recognition, self-driving cars, and text parsing. Text parsing is the angle where DCL uses CV. When we convert PDFs to XML, a form of CV is employed to scan the image-based PDFs, partition the page, and our algorithms take this data and transform it into the targeted XML structure. Obviously, it’s a lot more involved but that’s the general overview.
You do a lot of R&D here at DCL, can you share an experiment that really blew your mind?
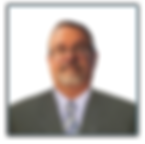
Rich Dominelli, Systems Architect, DCL
We did a prototype video analysis tool that analyzed a video deposition and showed the person's emotional state and heart rate. The emotional state was based on micro expressions as analyzed by a Microsoft tool kit. The heart rate was determined based on enhanced computer vision analysis of the persons changing skin tone.
Can you detail how DCL ensures our work with LLMs is safe, impartial, and verified?
Tammy Bilitzky, CIO, DCL
We know firsthand the value of accurate, high-quality training data. I would say we spend significant amounts of time and effort to cull through all of our data repositories and public information and make sure that the data we're using to train our LLMs has been vetted and verified. I venture to say we probably spend an equal amount of effort on creating the training data as a combination of everything else in our pipeline. Because we've heard these horror stories that stem from incorrect training data.
What is one of DCL’s experiments with AI that excites you?
Mark Gross, President, DCL
The future of AI is certainly captivating and exciting with things like autonomous vehicles, healthcare diagnostics, robotics, and so on, but I’m a pragmatic person and find value working on some of the “little things.” As an example, we have the technology to put people on the moon, but we still have challenges automatically structuring tables! DCL is changing this.
Transforming tabular content into a structured model such as XML or HTML is still often a manual or semi-manual process. Tabular content is particularly important in regulatory, financial, and scientific documents where complex alphanumeric content is often presented in tabular format. Tables are tough to structure due to inconsistencies with tabular content, high diversity of layouts, complicated elements such as straddle headings, various alignments of contents, the presence of empty cells, and other intricacies. While not as sexy as autonomous vehicles, creating an AI model that finds and extracts information from all tables in a document uses a combination of Computer Vision (CV), Natural Language Processing (NLP), and other AI technologies and is surprisingly difficult to do well. We've had great success with automated table conversions over the past year.
Another model we’ve been perfecting is a deep learning model that analyzes a page to detect equations automatically. DCL works with many scientific publishers who have seriously complex math in their journal articles. We’ve developed a process that can identify an equation that’s been produced as an image. Then, we convert the image and structure it into MathML, allowing automated equation generation from the XML.
Representing complex equations with MathML rather than displaying them as images offers the advantage of enhanced accessibility. MathML is machine-readable and supports assistive technologies, making mathematical content more inclusive for users with disabilities. Also, MathML allows for dynamic rendering, enabling responsive design and better adaptation to different devices and screen sizes, providing a versatile and user-friendly experience.
These are the kind of puzzles I like DCL to solve. We constantly speak with customers about struggles they have with content and data and would love to hear from you if you have a complex challenge. It’s very likely we can help!